Unlocking Business Potential with Data Labeling and Machine Learning
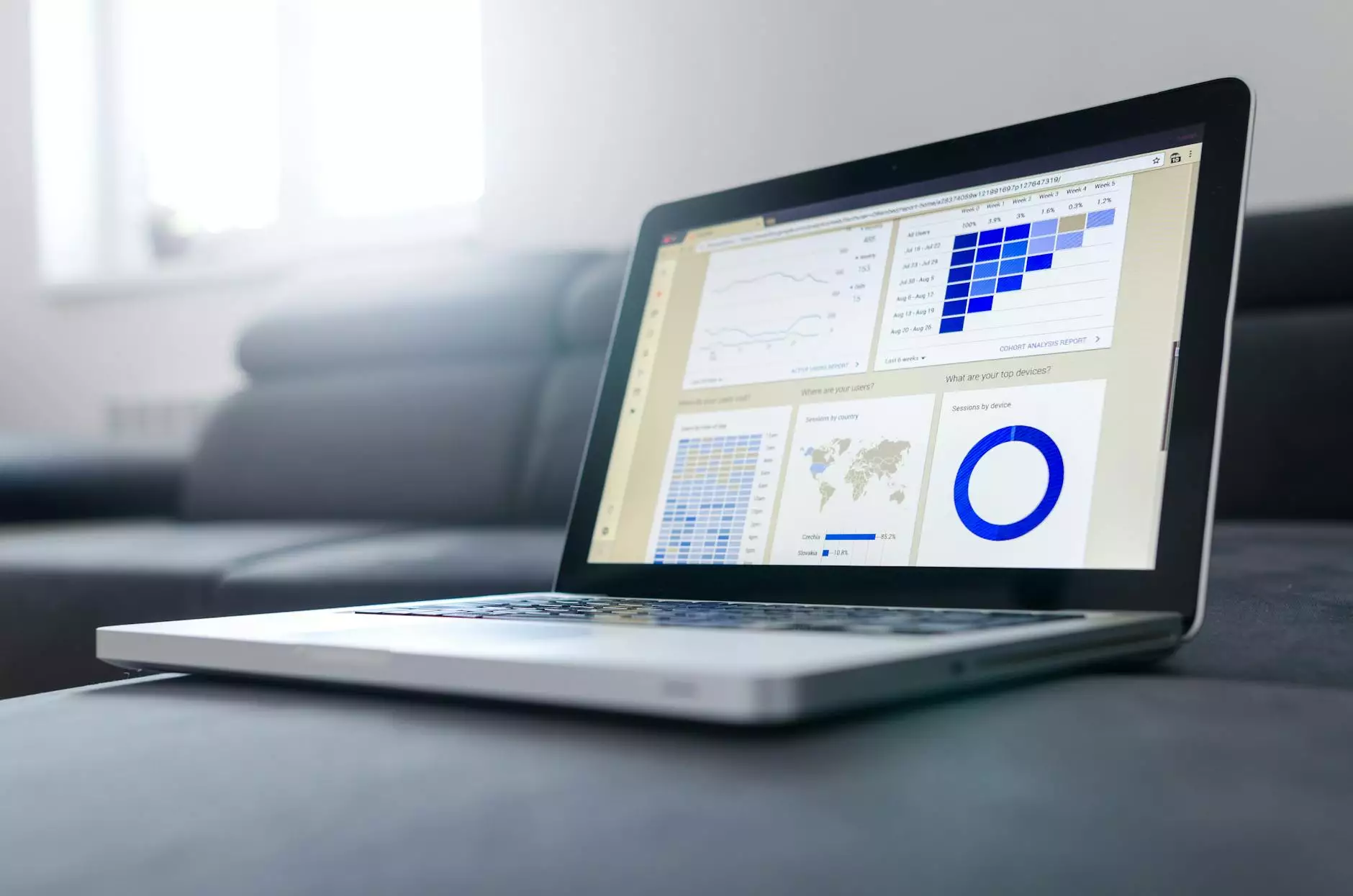
In the rapidly evolving world of technology, data labeling machine learning has emerged as a game-changer for businesses looking to harness the power of artificial intelligence. This article explores the pivotal role that data annotation plays in enhancing machine learning models, making it an invaluable asset for any modern enterprise. Join us as we delve into the intricacies of data annotation tools and platforms, and how they can supercharge your business strategies.
Understanding Data Labeling in the Machine Learning Ecosystem
Data labeling is the process of tagging or annotating data to make it understandable for machine learning algorithms. In essence, it transforms raw data into structured information, enabling machines to learn from it effectively. This is particularly crucial for supervised learning models, where accurate labeling is key to training algorithms to make predictions or classifications.
Why Data Labeling is Essential for Your Business
Businesses today rely heavily on data to drive insights and inform decision-making. The significance of data labeling machine learning can be summarized in several critical points:
- Improved Accuracy: Well-labeled data ensures that machine learning models perform with higher precision.
- Faster Model Training: Quality annotations accelerate the training process, allowing for quicker deployment of models.
- Customization: Tailored annotation processes can capture the unique requirements of your business's data.
- Enhanced Insights: Accurate data leads to better analytics, giving businesses deeper insights into customer behavior.
- Scalability: As your data grows, a robust labeling process aids in maintaining quality across large datasets.
Types of Data Annotation Techniques
Within the realm of data labeling, there are various techniques, each suited for specific types of data and applications:
1. Image Annotation
This involves labeling images for computer vision tasks. Techniques include:
- Bounding Boxes: Drawing rectangles around objects in an image to identify them.
- Semantic Segmentation: Classifying each pixel in an image to assign labels to distinguish different objects.
- Polygon Annotation: Defining the shape of objects more precisely using polygons.
2. Text Annotation
Text data requires annotation for natural language processing (NLP) tasks. Common methods include:
- Entity Recognition: Identifying entities such as names, dates, and locations within a text.
- Sentiment Analysis: Classifying the sentiment conveyed in a text, whether positive, negative, or neutral.
- Part-of-Speech Tagging: Labeling words with their respective parts of speech like nouns, verbs, etc.
3. Audio Annotation
For machine learning models that process audio, accurate labeling is vital. Techniques include:
- Transcription: Converting spoken words into written text.
- Labeling Events: Identifying specific sounds or events in an audio clip.
Choosing the Right Data Annotation Tools
When it comes to selecting data annotation tools, businesses need to consider several factors to ensure they are equipped for success:
- Scalability: The tool should easily scale to handle increasing volumes of data.
- Ease of Use: A user-friendly interface will expedite the annotation process.
- Collaboration Features: Look for tools that offer collaboration capabilities for teams to work together efficiently.
- Cost-effectiveness: Analyze the pricing model to ensure it aligns with your budget without compromising quality.
- Support and Training: Adequate support and resources can enhance the efficiency of your labeling process.
Keylabs.ai: Redefining Data Annotation for Businesses
At Keylabs.ai, we specialize in providing cutting-edge data annotation tools and platforms that empower businesses to leverage the power of machine learning.
The Key Features of Keylabs.ai
Our platform offers a suite of features designed to streamline the data annotation process:
- Automated Annotation: Leveraging AI to reduce manual effort and increase efficiency.
- High Accuracy Rates: Our trained annotators ensure consistent quality across all labeled datasets.
- Custom Solutions: Tailor our tools to meet your specific business needs and objectives.
- Real-time Collaboration: Integrated tools allow your team to work together seamlessly.
- Comprehensive Support: Our support team is available 24/7 to assist with any queries or issues.
Case Studies: Success Stories with Keylabs.ai
The impact of our data annotation platform can be illustrated through various success stories:
1. E-commerce Company: Enhancing Product Categorization
An e-commerce company struggled with categorizing products based on user-generated images. By utilizing our image annotation services, they improved their categorization accuracy and saw a 30% increase in user engagement on their platform.
2. Healthcare Sector: Improving Diagnostic Tools
A healthcare provider needed labeled medical images to train their diagnostic AI tool. With our support, they were able to compile a database that significantly enhanced their diagnostic accuracy, leading to better patient outcomes and a reduction in misdiagnoses.
3. Financial Services: Enhancing Fraud Detection
A financial institution utilized our text annotation services to improve their fraud detection systems. By accurately tagging transactions and customer interactions, they were able to reduce fraudulent activities by 40%.
The Future of Data Labeling in Machine Learning
The future of data labeling machine learning is promising. As businesses continue to recognize the value of machine learning, the demand for efficient, accurate, and scalable annotation tools will only grow. Trends emerging in this space include:
- Increased Automation: More sophisticated AI tools will democratize data labeling, allowing businesses to label data faster and with less human intervention.
- Integration with ML Pipelines: Annotation tools will increasingly integrate seamlessly with machine learning workflows, minimizing bottlenecks.
- Sophisticated Quality Control: Enhanced algorithms will provide better quality checks on labeled data, improving overall model performance.
Conclusion: Invest in Data Annotation for Long-Term Success
In conclusion, investing in data labeling machine learning is not just a strategic decision; it's a necessity in today's data-driven business landscape. By partnering with a reliable provider like Keylabs.ai, you can ensure that your data annotation processes are efficient, scalable, and aligned with your business goals.
As you move forward, remember that the quality of your data directly impacts the performance of your machine learning models. Choose wisely, and watch your business transform as you unlock the full potential of your data.